Transformers:
Single Architecture for Multiple Modalities





Evolution of How Information is Stored and Retrieved !
Stone/Iron Age
Industrial Age
Digital Age
Carved in Stones
Written on papers
Digitized
Parameterized


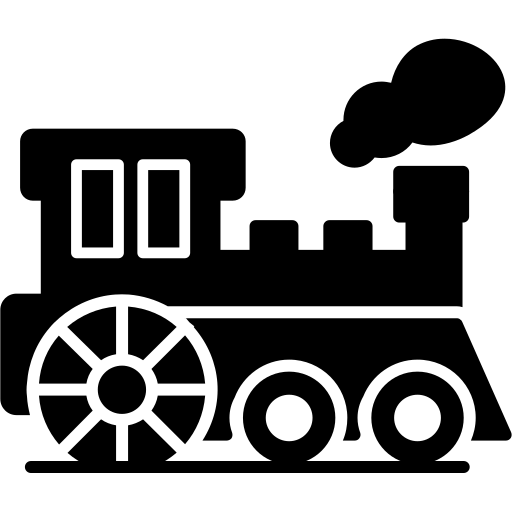

The Age of AI [has begun]*
Store and Retrieve
Store and Retrieve
Store and Retrieve
Store and Generate!








Generations of Language Modelling


1990

2013

Transformers
2017
Statistical LM's
Specific Task Helper
n-gram models
Task-agnostic Feature Learners
word2vec, context modelling
Neural LMs
Transfer Learning for NLP
ELMO, GPT, BERT
(pre-train,fine-tune)

LLMs
2020
General Language Models
GPT-3, InstructGPT
(emerging abilities)
Task solving Capacity


Magic Box





Creative Text Generation
"Any sufficiently Advanced Technology is Indistinguishable from Magic"







Simple Sentiment Classification
Magic Box
"Any sufficiently Advanced Technology is Indistinguishable from Magic"







Logical Reasoning
Magic Box
"Any sufficiently Advanced Technology is Indistinguishable from Magic"





Doing arithmetic


Magic Box
"Any sufficiently Advanced Technology is Indistinguishable from Magic"
Who is inside the Magic box?

I'm sure there must be a few expert dwarves in the box!
That's why we get convincing responses for all questions






Magic Box

"Any sufficiently Advanced Technology is indistinguishable from Magic"





Magic Box

Multi-head Masked Attention

tell
me
a
joke
about
idli




why
why




did
the
did
Multi-head Masked Attention
tell
me
a
joke
about
idli



why
why




did
the
did
The Magic:
Train the models to predict next word given all previous words


idli
the
“The magician takes the ordinary something and makes it do something extraordinary.”
Traditional NLP Models
Large Language Models
Input text
Predict the class/sentiment
Input text
Summarize
Question
Answer
Input text





LLMs
Prompt: Input text
Output response conditioned on prompt
Prompt: Predict sentiment, summarize, fill in the blank, generate story
Labelled data for task-1
Labelled data for task-2
Labelled data for task-3


Raw text data
(cleaned)
Model-1
Model-2
Model-3








Trillions of
Tokens
Billions of
Parameters
Zetta FLOPS
of Compute
LLMs
Three Stages
Pre-training
Fine tuning
Inference
Trident of LLMs
Trillions of Tokens





LLMs


Next token
“The magician takes the ordinary something and makes it do something extraordinary.”
Something Ordinary:
To Extraordinary:
Predict next token
and next token, next token, .........
Sourcing billions of tokens from the Internet is a massive engineering effort!!
Pre-Training
By doing this, the model eventually learns language structure, grammar and world knowledge !
Trillions of
Tokens
BookCorpus
Wikipedia
WebText(closed)
RealNews
The Pile
ROOTS
Falcon
RedPajama
DOLMA
C4
Opportunity:
Build one
Challenge:
Inadequate quality datasets for Indic Languages
Effort by AI4Bharat



English data
Capture all India specific knowledge in all Indian Languages!


Billions of
Parameters


Fruit Fly
Honey Bee
Mouse
Cat
Brain
# Synapses
Transformer
GPT-2
Megatron LM
GPT-3
GShard



Zetta FLOPS
of Compute

Training LLMs having more than 70 Billion Parameters is affordable only for a few organizations around the world
Requires a cluster of A100 (or) H100 GPUs that requires millions of dollars
Then, how do we adapt those models for diverse Indian culture and languages
Way to go: Language Adaptation ?






Trillions of
Tokens
Billions of
Parameters
Zetta FLOPS
of Compute
Pre-Trained open sourced LLM


Way to go: Language Adaptation ?





Trillions of
Tokens
Billions of
Parameters
Zetta FLOPS
of Compute
Pre-Trained open sourced LLM


Billions of
Tokens
Billions of
Parameters
Peta FLOPS
of Compute
Fully fine-tuned
Sangraha




Billions of
Parameters


Fruit Fly
Honey Bee
Mouse
Cat
Brain
# Synapses
Transformer
GPT-2
Megatron LM
GPT-3
GShard
Affordable for inference
Opportunity:
Use Instruction Fine-tuning and build datasets for the same
Challenge:
(full) Fine-Tuning of LLMs on Indic datasets still requires a lot of compute and expensive
Way to go: Instruction Fine-Tuning

Millions of
Tokens
Billions of
Parameters
Tera FLOPS
of Compute
Instruction-tuned
Indic-Align



Goal:
Improve the model’s ability to understand and follow human instructions and ensure response is aligned with human expectations and values.
How it works:
Training the model on a set (relatively small) of high quality and diverse instruction and answer pairs.
The Transformer
A simple encoder-decoder model with attention mechanism at its core
Takes in a sequence [of words:Tokens:Embeddings]
Outputs a sequence [conditional probabilities: predicted tokens]
Let's first understand the input block
Source Input Block
Target Input Block
Output Block (tied)
Multi-Head Attention
Feed forward NN
Add&Norm
Add&Norm
Multi-Head cross Attention
Feed forward NN
Add&Norm
Add&Norm
Multi-Head Masked Attention
Add&Norm
The Transformer
A simple encoder-decoder model with attention mechanism at its core
Encoder
Decoder
I am reading a book
Naan oru puthagathai padiththu kondirukiren
Naan oru puthagathai padiththu kondirukiren
Source Input Block
Target Input Block
Input Block
Tokenizer
Token Ids
embeddings
I am reading a book
Input Block
Tokenizer
I am reading a book
["i, am, reading, a, book]
Contains:
- Normalizer
- Pre-tokenizer
- tokenization algorithm
Normalizer: Lowercase, (I ->i)
Pre-tokenizer: Whitespace
Tok-algorithm: BPE
-
We have to train the tokenization algorithm using all the samples from a dataset
-
It constructs a vocabulary of size \(|V|\) (Typically, 30000+, 50000+ )
-
Then the tokenizer splits the input sequence into tokens (token could be a whole word or a sub-word)
Input Block
Tokenizer
I am reading a book
["i, am, reading, a, book]
-
Each token is assigned with a unique integer (Id)
-
These IDs are unique to tokenizers used trained on a particular dataset
-
Therefore, we have to use the same tokenizer (used to train a model) for all downstream tasks
Token Ids
["i:2, am:8, reading:75, a:4, book:100]
-
Model-specific special tokens are inserted into the existing list of token_ids, for example
["[BOS]:1, i:2, am:8, reading:75, a:4, book:100,[EOS]:3]
Input Block
I am reading a book
[" [BOS]:1 i:2 am:8 reading:75a:4 book:100 [EOS]:0 ]
Tokenizer
Token Ids
embeddings
-
Embedding is a look-up table that returns a vector of size, say: 512,1024,2048..
-
The token "i" is assigned a vector at index 2 in the embedding table
-
The token "a" is assigned a vector at index 4 in the embedding table
-
This mapping goes on for all the tokens in an input sequence
-
All the embedding vectors are LEARNABLE
Input Block
I am reading a book
[" [BOS]:1 i:2 am:8 reading:75a:4 book:100 [EOS]:0 ]
Tokenizer
Token Ids
embeddings
-
We have another embedding table to encode position of tokens [learnable or fixed]
position embeddings
-
We add these position embeddings to the corresponding token embeddings
-
The number of position embeddings depends on the context (window) length of model
-
THE ENTIRE PROCESS IS REPEATED FOR TARGET INPUT BLOCK
I am reading a book
Input Block
Source Input Block
Embedding for each input token
-
The embedding vectors are randomly initialized
-
Along with these we also pass in attention mask, padding mask for batch processing
Multi-Head Attention
Feed forward NN
Add&Norm
Add&Norm
Multi-Head cross Attention
Feed forward NN
Add&Norm
Add&Norm
Multi-Head Masked Attention
Add&Norm
-
The encoder and decoder of the transformer blocks takes in embedding vectors as input and produces output probability over tokens in the vocabulary

reading
I
am
a
book
Naan
puthakathai
padithtu
kondirukiren
Configuration
One can construct a transformer architecture given the configuration file with the following fields
- \(d_{model}\): model dimension(=embedding dimension)
- \(n_{heads}\):Number of heads
- \(dff\): Hidden dimension (often, \(d_{ff}=4d_{model}\))
- \(n_{layers}\): Number of layers
- \(droput_{prob}\): for Feed Forward, Attention, embedding
- activation function
- tie weights?
The Transformer
Multi-Head Attention
Feed forward NN
Add&Norm
Add&Norm
Multi-Head cross Attention
Feed forward NN
Add&Norm
Add&Norm
Multi-Head Masked Attention
Add&Norm
Originally Proposed for Machine Translation task
The field has evolved rapidly since then in multiple directions
-
Improvements in attention mechanisms, positional encoding techniques and so on
-
Scaling the size of the model (parameters, datasets, training steps)
-
Extensive studies on the choices of hyperparameters
Dataset
Source Input Block
Target Input Block
Architectural Improvements
Multi-Head Attention
Feed forward NN
Add&Norm
Add&Norm
Multi-Head cross Attention
Feed forward NN
Add&Norm
Add&Norm
Multi-Head Masked Attention
Add&Norm
Position Encoding
- Absolute
- Relative
- RoPE,NoPE
- AliBi
Attention
- Full/sparse
- Multi/grouped query
- Flashed
- Paged
Normalization
- Layer norm
- RMS norm
- DeepNorm
Activation
- ReLU
- GeLU
- Swish
- SwiGLU
- GeGLU
- FFN
- MoE
- PostNorm
- Pre-Norm
- Sandwich
Pre-training and Fine-Tuning
Preparing labelled data for each task is laborious and costly
On the other hand,
We have a large amount of unlabelled text easily available on the internet
Transformer
Transformer
Transformer
Input text
Predict the class/sentiment
Input text
Summarize
Question
Answer
Input text


Can we make use of such unlabelled data to train a model?
That is called pre-training.
However, what should be the training objective?
Pre-training Objectives
Encoder
Decoder
Multi-Head Attention
Feed forward NN
Add&Norm
Add&Norm
\(x_1,<mask>,\cdots,x_{T}\)
\(P(x_2=?)\)
Multi-Head masked Attention
Feed forward NN
Add&Norm
Add&Norm
\(x_1,x_2,\cdots,x_{i-1}\)
\(P(x_i)\)
Multi-Head Attention
Feed forward NN
Add&Norm
Add&Norm
Multi-Head cross Attention
Feed forward NN
Add&Norm
Add&Norm
Multi-Head Maksed Attention
Add&Norm
\(x_1,<mask>,\cdots,x_{T}\)
\(<go>\)
\(.,P(x_2|x_1,).,\)
Encoder-Decoder
Objective: MLM
Objective: CLM
Objective: PLM,Denoising
Example: BERT
Example: GPT
Example: BART,T5
Generative Pre-trained model (GPT)
Pre-training Objective: CLM
Transformer Block 1
Transformer Block 2
Transformer Block 3
Transformer Block 4
Transformer Block 5




Assume that we have now (pre)trained a model.
Now, we can fine-tune it on different tasks (with slight modifications in the output head) and put it for inference
Causal mask allows us to compute all
these probabilities in a single go
Decoding Strategies
Beam Search
Top-P (Nucleus Sampling )
Greedy Search
Top-K sampling
Deterministic
Stochastic
Suitable for translation
Suitable for tasks like Text generation, summarization
BERT: MLM
[mask]
enjoyed
the
[mask]
transformers
Encoder Layer (attention,FFN,Normalization,Residual connection)
Encoder Layer (attention,FFN,Normalization,Residual connection)
Encoder Layer (attention,FFN,Normalization,Residual connection)




A multi-layer bidirectional transformer encoder architecture.
BERT Base model contains 12 layers with 12 Attention heads per layer
The masked words (15%) in an input sequence are sampled uniformly
Of these, 80% are replaced with [mask] token and 10% are replaced with random words and remaining 10% are retained as it is. (Why?)
Is pre-training objective of MLM sufficient for downstream tasks like Question-Answering where interaction between sentences is important?
the special mask token won't be a part of the dataset while adapting for downstream tasks
Now, let's extend the input with a pair of sentences (A,B) and the label that indicates whether the sentence B naturally follows sentence A.
[CLS]
I
enjoyed
the
movie
Feed Forward Network
Self-Attention
transformers
[SEP]
The
visuals
were
amazing
input: Sentence: A


input: Sentence: B
Label: IsNext
Next Sentence Prediction(NSP)
Special tokens: [CLS],[SEP]
[CLS]
I
enjoyed
the
movie
transformers
[SEP]
The
visuals
were
amazing
Position Embeddings
Segment Embeddings
Token Embeddings
Feed Forward Network
Self-Attention


Encoder Layer
Encoder Layer
Encoder Layer
Encoder Layer
[CLS]
[mask]
enjoyed
the
[mask]
transformers
[SEP]
The
[mask]
were
amazing








Minimize the objective:
What is the best setting?
encoder
encoder
decoder
decoder
Scale:
- small
- medium
- large
Objective:MLM
- corruption rate
- token deletion
- span mask
Scale:
- small
- medium
- large
Scale:
- small
- medium
- large
pre-Training
pre-Training
pre-Training
Pretraining:
- Wiki
- Books
- Web Crawl
Fine-Tuning
Fine-Tuning
Fine-Tuning
FineTuning:
- GLUE
- SQUAD
- SuperGLUE
- WMT-14
- WMT-15
- WMT-16
- CNN/DM



hyp-params:
- num.of train steps
- learning rate scheme
- optimizer
hyp-params:
- num.of train steps
- learning rate scheme
- optimizer
hyp-params:
- num.of train steps
- learning rate scheme
- optimizer
Objective:
- de-noising
- corrpution rate
- continous masking
FineTuning:
- GLUE
- SQUAD
- SuperGLUE
- WMT-14
- WMT-15
- WMT-16
- CNN/DM
Objective:
- CLM
- prefix-LM
- conditional
FineTuning:
- GLUE
- SQUAD
- SuperGLUE
- WMT-14
- WMT-15
- WMT-16
- CNN/DM
Language Models are Few Shot Learners, conditional learners
Text to text framework
Instruction fine-tuning
Aligning with user intent
Retrieval Augmented Generation
Agents
Hugging Face Is Here to Help us

Transformer-is-All-you-Need
By Arun Prakash
Transformer-is-All-you-Need
- 231